SciML is working closely with ISIS neutron and muon source on a number of projects covering the range from controlling the injection of beam to the synchrotron all the way to analysing the final data to discover new science. Below we have a selection of the on-going/completed projects to give a flavour of the work that we are doing with ISIS.
Data analysis
We have projects with a number of different beamlines in ISIS, covering areas such as diffraction, small angle scattering and inelastic scattering. We have helped to develop tools that allow scientists to work more efficiently and analyse data in a way that was not possible before.
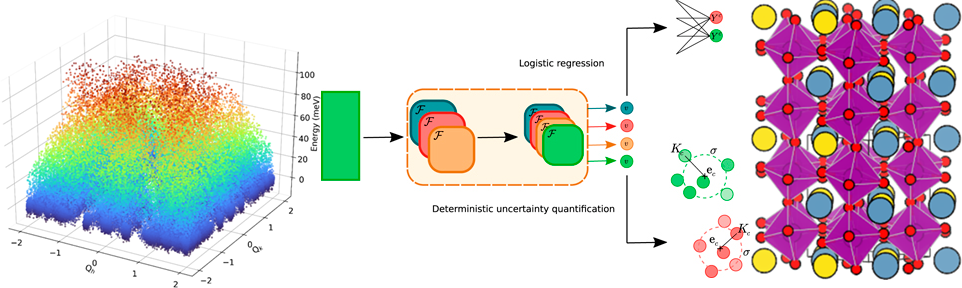
![]()
For Small Angle Scattering (SAS) SciML has worked with scientists at ISIS and Diamond to develop a free-form SAS solution tool. FFSAS allows users to solve SAS signals and obtain distributions of scatters from the data. The typical inverse problem of SAS has been formulated as a solvable non-linear programming problems and highly accurate predictions of system distribution in a fraction of the time required by the existing gold-standard sampling approaches.
For neutron diffraction we have been helping to develop efficient algorithms for identifying interesting peaks in complex spectra. Examples of use cases are metal organic frameworks and thin film systems, where background peaks from sample holders and substrates often dominate the signal and make identification of peaks of interest extremely difficult. Our 3D morphology-based algorithm can detect most of the Bragg peaks even in a highly noisy and inhomogeneous background, with an axillary algorithm to screen out false positives. Our tests show that it can detect Bragg peaks hardly visible by eyes, and thus outperform human detection.
In inelastic neutron scattering (INS) we have helped to tackle the problem of solving magnetic structures from magnon spectra. Typically magnon spectra are complex 3 or 4-D datasets where very small regions of the spectrum can be very important for determining the structure, generally necessitating extensive manual searching. In this project we have developed neural networks that can classify structures based on a magnon spectrum, but then can also identify the region or regions of the spectrum which are important for that classification – thus acting a companion to guide scientists in their search through complex data. You can read more about this work in our recent paper Interpretable, calibrated neural networks for analysis and understanding of inelastic neutron scattering data.
Synchrotron Control
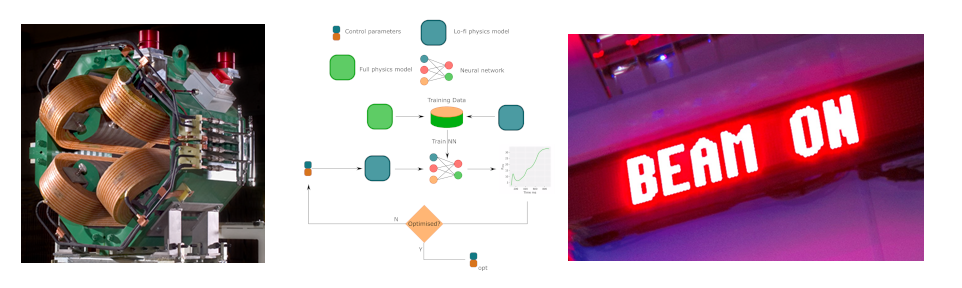
We are working with the ISIS controls team to develop machine learning (ML) tools to help with the optimisation of beam injector profiles. Ultimately the aim is to produce a more concentrated beam which strikes the foil less often. Currently this kind of optimisation is done based on physics based models, but there is a trade-off; the very accurate models are too expensive to use for iterative optimisation, while the quicker models are not accurate enough to trust the results. We have produced a ML model that is capable of producing results of comparable accuracy to the accurate physics mode, but in greatly reduced time (12 hrs to 2 sec), facilitating optimisation procedures (e.g. genetic algorithms) to be used to optimise the input parameter profiles. This tool is ultimately suitable for any synchrotron and can be used to greatly enhance the efficiency of running expensive beamlines.